The Limitations and Challenges of Fluid Artificial Intelligence
Updated: June 19, 2024
Published: May 5, 2022
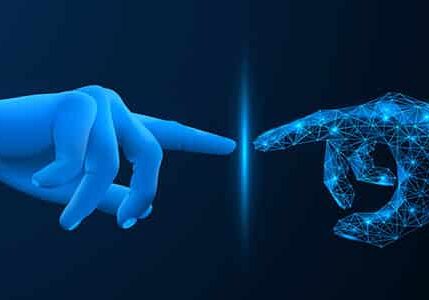
Futurists have prognosticated the replacement of humans with robots, powered by artificial intelligence, for at least six decades. While computers are far more efficient than humans at crystalized tasks, such as information recall, pattern recognition, and statistical inference, they are far worse than humans in demonstrating, ‘fluid intelligence’, or operating independently of rules, processing new information, or performing tasks without clear objectives.
The concept of fluid versus crystallized intelligence dates to 1963, when it was introduced by one of the most influential psychologists of the 20th century, Raymond Cattell (1905-1998). Fluid intelligence is the ability to think flexibly, reason, and process novel information in real time. In contrast, crystallized intelligence refers to knowledge gained from prior learning of facts, skills, and experiences (Psychology Today).
In 2003, Marshall Brain claimed, “over the next 40 years robots will replace most human jobs. According to Brain’s projections, in his essay ‘Robot Nation’, humanoid robots will be widely available by 2030…Brain estimates more than 50% of Americans could be unemployed by 2055 – replaced by robots” (Pinto 2003). Similarly, the World Future Society forecasted in 2007 that employees will be less valuable and compensation would decrease as humans would now face competition from machines, as, “businesses will hire whatever type of mind can do the work—robotic or human” (SHRM). Yet 20 years since Brain’s prediction there is little evidence of automation causing widespread unemployment. In fact, even during a global pandemic, American businesses are struggling to find enough workers, lowering production, limiting hours of operation, and increasing prices (CNN).
The development of computer processing power has followed Moore’s Law since 1970, as the number of transistors on integrated circuits has (more than) doubled every two years. Today, even consumer chips, like Apple’s M1 Max, have well over 50 billion transistors. The capabilities of these machines to both run incredibly complicated programs as well as compute exponentially more tasks and calculations than a human, however, has not resulted in comparable gains for a machine’s fluid intelligence. Those who have attempted to use a voice device like Amazon’s Alexa or Google’s Home products know even the most basic commands or requests can be incredibly frustrating. Moreover, a substantial roadblock for researchers in developing machine fluid intelligence is that humans themselves are unable to convert their fluid intelligence to a ‘higher’ form. For instance, if increasing someone’s I.Q. were an activity like solving a set of math puzzles, we ought to see successful examples of it at the low end, where the problems are easier to solve. But we don’t see strong evidence of that happening (Chiang).
While increasing intelligence in humans is remarkably difficult, even the most sophisticated models, continuously fed with nearly-limitless datasets, fail to prove to be adequate replacements for humans in utilizing fluid intelligence. Indeed, just because humans can solve difficult problems requiring fluid intelligence does not mean humans can describe, let alone replicate, the mechanisms underlying decision-making that are defined as ‘fluid intelligence.’
Consider some of the tasks at which A.I. programs currently excel: recalling information (such as IBM’s Deep Blue), identifying patterns (such as facial recognition technology and autonomous driving), robotics (improving efficiencies of factory robots), and online advertising (recommendation engines that power Google’s Ad Services and Netflix’s recommendations). Each of these is both highly focused on a defined outcome (recalling accurate information to a given question and subject to rules constructed by humans.
Perhaps the most prevalent integration of AI in the lives of most people is through the ‘internet of things’, traditional devices from refrigerators to vacuum cleaners to thermostats that, by being connected to the internet, can learn the patterns and habits of their owners and adapt their behavior accordingly (European Parliament, 2021). Learning our patterns makes these machines appear more ‘human’, yet it is only with being provided a dataset (in this case, human behaviors) that they can adapt their behaviors. Fluid intelligence, on the other hand, would require these machines to make decisions given incomplete datasets, making decisions under uncertain conditions.
According to the U.S. Office of Naval Research, extraordinary advances in computing power make computers appear to be nearing fluid intelligence but instead are only engaging in, “task-transductive mechanisms” that increase the efficiency of computers performing tasks of ‘crystalized intelligence, relying on, “accessing problem-specific knowledge, skills, and experience stored in long term memory” (Davidson 2019). Artificial intelligence and machine learning increase the efficiency with which machines perform tasks, yet those tasks haven’t substantially changed since Charles Babbage’s Difference Engine in 1822.
Machines make humans more intelligent by performing tasks that would otherwise require substantial time, energy, and training. Exponential advances in computing power democratize information processing, allowing billions of people access to tools that relieve otherwise insurmountable obstacles to data processing and management. These tools themselves, however, do not make humans more intelligent, and cannot demonstrate fluid intelligence, let alone make themselves more ‘intelligent’ (distinct from making themselves more efficient) without human intervention and direction. Ted Chiang summarizes the predicament, “In the same way that we needn’t worry about a superhumanly intelligent A.I. destroying civilization, we shouldn’t look forward to a superhumanly intelligent A.I. saving us in spite of ourselves. For better or worse, the fate of our species will depend on human decision-making. (Chiang)